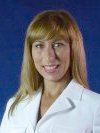
Energy-Efficient Distributed Signal Processing in Mobile Wireless Sensor Networks
Dr. Loredana Arienzo
Institute for the Protection and Security of the Citizen Joint Research Center, European Commission
Abstract
Wireless mobile sensor networks are important for a number of strategic applications such as surveillance, fire detection, outlier detection. Energy is a critical resource in wireless sensor networks and system lifetime needs to be prolonged through the use of energy-efficient signal processing during system operation. In this talk an overview of statistical prediction frameworks for tracking dynamic targets in range-based signal processing is presented. The single mobile target algorithm has been evaluated by the metrics of tracking precision and network energy consumption. The computation of the posterior Cramer-Rao bound (PCRB) for range-based target tracking has been considered. PCRB is a theoretical lower bound on the estimation error while assessing the performance of any kind of estimation algorithm. Here the method is applied to a nonlinear filtering problem of tracking node in wireless sensor networks. The evaluation is performed using the constant velocity model and the path loss propagation model, respectively, as dynamic model and measurement model. The bound is computed against the root mean square error of two non linear filters: bootstrap and unscented particle filter. A novel tradeoff between the accuracy of the estimation bound and the energy consumption is also showed. The tutorial will conclude by discussing distributed data fusion algorithms based upon a Bayesian approach.
Biography
Loredana Arienzo is a researcher at the Institute for the Protection and the Security of the Citizen in the Joint Research Centre of European Commission since December 2008. She received her PhD in Information Engineering from the University of Salerno in 2008. Loredana Arienzo was a visiting researcher in BWN Lab, School of Electrical and Computer Engineering, Georgia Institute of Technology, Atlanta, in 2006. She received her B.Sc. and M.Sc. degree in Electronics Engineering from the University of Salerno (IT), in 1999 and her Associate degree in Software Engineering from the University of Benevento (IT), in 2001. Her research interests focus on wireless communications, ad-hoc and sensor networks, distributed signal processing, tracking in ad-hoc and wireless sensor networks, cognitive radio networks. She is part of the European Concerted Research Action IC0902: Cognitive Radio and Networking for Cooperative Coexistence of Heterogeneous Wireless Networks. Loredana Arienzo is a member of IEEE, IEEE Communication Society and ACM. She is a reviewer for IEEE Transactions on Vehicular Technology, IEEE Communications Letters and 2010 IARIA Advanced International Conference on Telecommunications.
Summary
Sensor networks are very attractive for reconnaissance and surveillance applications as they provide more information from the same target, which helps to identify, classify, and track it. Furthermore, sensor networks possess a higher probability to survive if individual sensors fail. The first part of the tutorial presents several aspects of sensor networks and illustrates various properties of distributed networks composed of identical sensors. Looking at a sensor network and its operation these can be divided into two main categories: mono-type or multi-type sensor networks. Networks composed of different sensor types allow determining various characteristics of the same target and therefore to improve the classification and identification task, but not necessarily the target localization accuracy. Depending on the arrangement of the sensors in the network a further classification can be introduced as centralized network or distributed sensor network. The advantage of a distributed mono-type sensor network is the possibility of bi-/multistatic operation. That implies, a receiver node is able to receive echoes from the target illuminated from different transmit nodes. Furthermore, spatially distributed monotype sensor networks offer more degrees of freedom than a system with one sensor. These multiple-input multiple output (MIMO) networks support flexible time-energy management modes, exhibit higher angular resolution, show an improved parameter estimation, and facilitate high resolution target localization accuracy with an improved detectability by exploiting target echo (RCS) diversity. An optimal MIMO network comprises multiple transmitters and multiple receivers to pick-up the reflected signal. Radar systems with their all-day, all weather ability are promising sensors for MIMO networks for surveillance applications in defense and civilian categories. On the contrary, if a network is composed of different sensor types each node can only operate in a monostatic configuration. Some issues of target localization for distributed MIMO radar networks are introduced. Due to their improved target parameter estimation capability distributed MIMO radar networks are very attractive for system designers. In particular their improved angular resolution capability and ability to separate multiple targets, to improve parameter identification, and to improve radar performance by exploiting radar cross section diversity is very attractive for surveillance applications. Likewise MIMO radar networks can handle slow moving targets by exploiting Doppler estimates from multiple directions and feature a highly accurate estimation of target position. Finally, the Position dilution of precision (PDOP) is introduced as a good tool for visualizing locating accuracy for a given multistatic radar network layout; it is originated with launching the Loran-C navigation system and came into much wider usage with GPS.
Energy is a critical resource in wireless sensor networks and system lifetime needs to be prolonged through the use of energy-efficient signal processing during system operation. In the second part of the tutorial an overview of statistical prediction frameworks for tracking dynamic targets in range-based signal processing is presented. The target tracking theory based on Bayesian estimation and estimator (MMSE, MMAE, MAP) is firstly introduced, then static and the dynamic target tracking problem is discussed. The single mobile target algorithm has been evaluated by the metrics of tracking precision and network energy consumption. We begin with a discussion of performance evaluation methods. The familiar CRLB (Cramer-Rao low bound) has been showed and the computation of the posterior Cramer-Rao bound (PCRB) for range-based target tracking has been considered. PCRB is a theoretical lower bound on the estimation error while assessing the performance of any kind of estimation algorithm. Here the method is applied to a nonlinear filtering problem of tracking node in wireless sensor networks. The evaluation is performed using the constant velocity model and the path loss propagation model, respectively, as dynamic model and measurement model. The bound is computed against the root mean square error of two non linear filters: bootstrap (PF) and unscented particle (UPF) filter. A novel tradeoff between the accuracy of the estimation bound and the energy consumption is also showed.
In the last part of the tutorial we discuss a number of concerns and items of interest that related to the tracking of targets in clutter. We begin with a discussion of performance evaluation methods. The familiar CRLB is shown to be adjusted in a straightforward way when deterministic trajectories are estimated in the presence of measurement-origin uncertainty (false alarms and missed detections); indeed, the same is true for nondeterministic ones, meaning those with process noise. We then mention the HYCA (hybrid conditional averaging) approach, which has considerable appeal in that it can predict the track-life as opposed to the accuracy of tracks that are kept. We then discuss track testing: the sequential probability ratio test (SPRT) for track acceptance, the Page test for track deletion, and here we most especially discuss variants (such as Shiryaev) for the case of fluctuating targets, as would be found in multi-static (fused) systems.
Multitarget tracking in clutter is a joint detection and estimation problem. It comprises two important inter-related tasks. One initiates and terminates targets, and the other associates, or assigns, data to specific targets and to clutter. The MHT (Multiple Hypothesis Tracking) formulation treats both tasks. It is well matched to the target physics and to the sensor signal processors of most radar and sonar systems. Unfortunately, exact MHT algorithms are intractable because the number of measurement assignment hypotheses grows exponentially with the number of measurements. These problems are aggravated when multiple sensors are used. Circumventing the computational difficulties of MHT requires approximation. The impact of a missed detection using the PHD (Probability hypothesis density) filter and the Cardinalized PHD (CPHD) filter are showed. In recent years the Probability Hypothesis Density (PHD) filter and the Cardinalized PHD (CPHD) filter have received increasing international attention. One of the assumptions underlying both filters is that all measurements are traditional, that is, a representation of conventional statistical measurement models and conventional likelihood functions. However, many measurements are non-traditional including attributes, features, natural-language statements, inference rules and more. This tutorial will describe the Bayesian random set approach to representing, fusing, and filtering such evidence. It will show that nontraditional evidence can be processed in essentially the same way as traditional evidence via statistically defined likelihood functions.